Higher Education
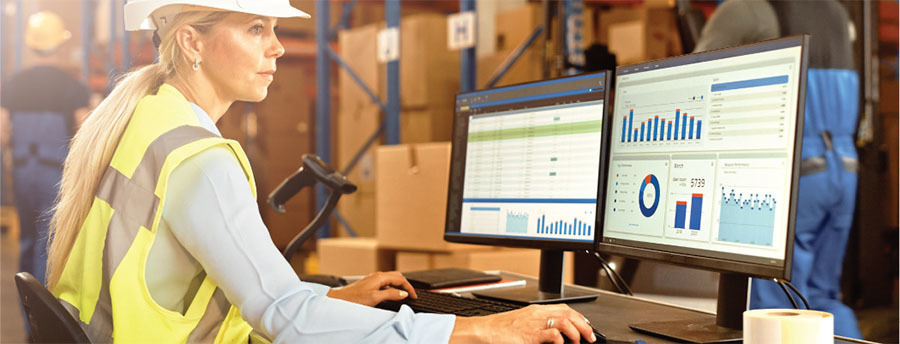
Advances in technology have made it easier for companies to amass extensive amounts of data about every aspect of their supply chain, in real-time. Using this technology in conjunction with artificial intelligence/machine learning (AI/ML) algorithms has resulted in supply chains that are “smart.” Smart implies that data and technology are leveraged to provide increased automation, situational awareness and advanced information about the potential supply chain disruptions. Consequently, supply chains that possess aspects of smart or intelligent technology can quickly adapt to shifting patterns in supply and demand.
A recent report by Gartner highlights some important trends with respect to supply chains of the future. First, technology provides strategic advantage, so it is important for business owners to digitize their supply chains. Digitizing the supply chain means moving away from disconnected systems and manual processes for tracking and tracing products. These actions limit the ability to respond with speed to shifting market demands, primarily due to the lack of real-time visibility to various aspects of the supply chain. Moving towards a digitization of processes is the first step towards obtaining a smart supply chain.
Secondly, supply chain management software is expected to incorporate more advanced analytics and AI to support human decision-making. The ability to interpret and take action on the data and recommendations generated from AI/ML is at the core of data-driven decision making. However, a key challenge associated with providing advanced analytic recommendations to the user is model interpretability. The unbalanced focus on the analytics by many researchers often resulted in complex models that are not easily understood by a human, and therefore, adding difficulties to the use of these models in support of decision making. The difficulty could be reflected by the lack of explanation of the cause of the decision made by the model as once said by Albert Einstein, “If you can’t explain it simply, you don’t understand it well enough” or lack of consistency in a human’s prediction of the model’s result.4 It is very important to recognize the constraints of human cognition and take human cognition into consideration when addressing model interpretability. Clearly, there is a gap between the complex model behavior and the human behavior that causes models to be less interpretable. One way to bridge that gap is through the use of data visualization.
Data visualization is a core data science approach that involves creating meaning out of data. There are a number of graphical tools that can be used such as bar plots, scatter plots, line plots and choropleth maps. For an extensive list, refer to [5]. Picking the right tool is not a trivial task. A number of factors must be considered, which are briefly summarized below:
- What are the key questions the data consumer (DC) will be trying to answer with the data?
- How will the DC desire to interact with the data?
- Which visual elements should be considered when adding multiple layers of information to a single chart? For example, are there standard colors that were used in spreadsheet graphs that denote meaning and should those colors be incorporated in any new visualizations of similar content?
- How should multiple visualizations be linked together to create a single, interpretable dashboard that facilitates exploration and decision-making?
Answers to these questions imply that the DC is closely involved in the design process, which is the essence of human-centered design (HCD). HCD is a methodology that puts the user at the center and incorporates their knowledge, work process and pain points into the design. It is an iterative, systematic process that involves analysis, design and evaluation methods to study users’ goals, task performance and preferences, as well as gaining feedback on design solutions.